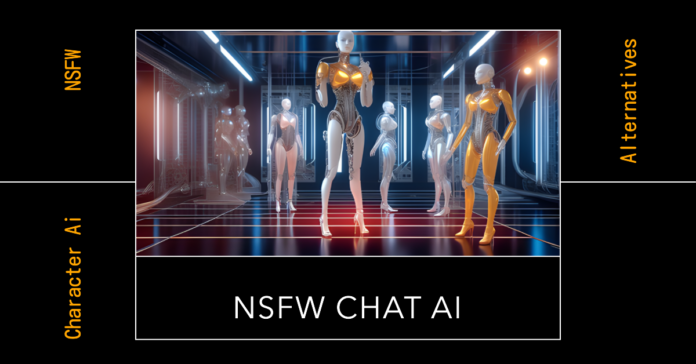
In the digital age, Not Safe For Work (NSFW) content has become a significant part of online communities and chat environments. This raises questions about the algorithms and technologies that govern these spaces.
This article explores the intricate mechanisms behind NSFW chats, shedding light on how they operate and the challenges they face in moderating content.
The Foundation of NSFW Chat Algorithms
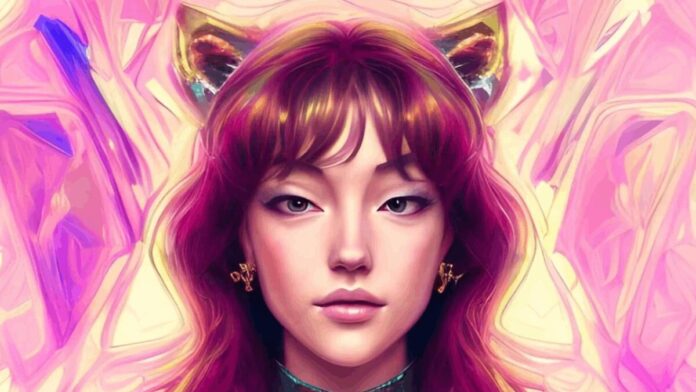
NSFW AI chat algorithms are designed to identify and manage content that is inappropriate for general audiences.
At the core, these systems use a combination of machine learning models and keyword filtering to scan text, images, and videos in real-time. This initial layer of detection is crucial for maintaining the integrity of the chat environment.
The algorithms are trained on vast datasets containing examples of both safe and unsafe content. This training enables them to discern subtle nuances in digital media, making them effective in filtering out explicit material.
However, the dynamic and evolving nature of language and imagery presents a continuous challenge, requiring constant updates and retraining of the models.
Beyond basic detection, advanced NSFW chat algorithms incorporate context analysis to understand the conversation’s flow.
This includes recognizing sarcasm, jokes, and idiomatic expressions, which are often challenging for machines. Such sophistication reduces false positives, where harmless content is mistakenly flagged as inappropriate.
Image and video analysis benefit from deep learning techniques, especially convolutional neural networks (CNNs), which can analyze visual content at a granular level.
These networks identify explicit content based on shape, color, texture, and other visual cues, distinguishing between acceptable and unacceptable imagery with high accuracy.
Challenges and Ethical Considerations
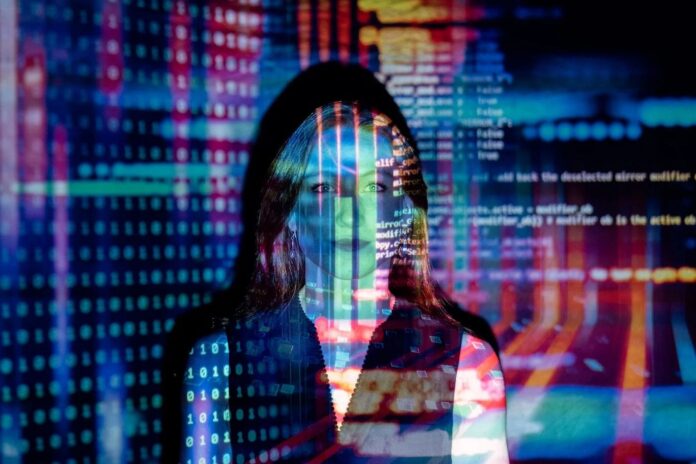
One of the main challenges in NSFW chat moderation is maintaining a balance between safeguarding users from harmful content and respecting freedom of expression.
Overly aggressive filters might censor legitimate conversations, stifling community engagement and expression. This delicate balance demands sophisticated algorithms that can interpret the intent and context of shared content.
Ethical considerations also come into play, particularly concerning privacy and censorship. Users expect a degree of privacy even in monitored environments, which necessitates transparent policies on data handling and moderation practices.
Ensuring that moderation algorithms are fair and unbiased is another critical aspect, as AI systems can inadvertently perpetuate biases present in their training data.
The digital landscape is continuously evolving, with new slang, symbols, and media formats emerging regularly.
NSFW chat algorithms must adapt to these changes to remain effective. This involves not only technical updates to the models but also a deeper understanding of cultural and social dynamics that influence online communication.
User feedback plays a vital role in refining these algorithms. Reporting mechanisms allow users to flag content that may have slipped through the filters, providing valuable data for improving the system.
Collaboration with experts in linguistics, psychology, and social sciences can also enhance the algorithm’s ability to navigate the complex web of human communication.
Conclusion
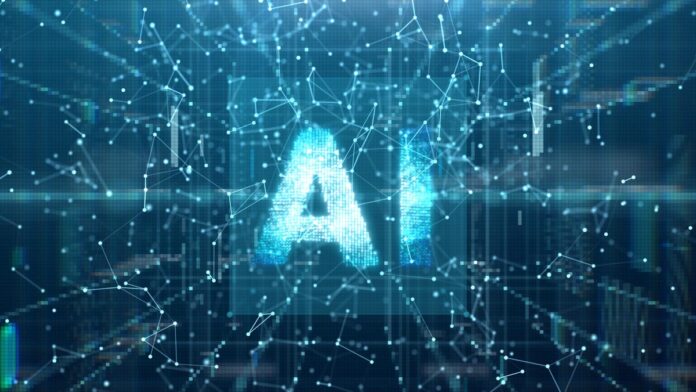
NSFW chat algorithms represent a complex interplay of technology, ethics, and social responsibility. While they are not perfect, ongoing advancements in AI and machine learning continue to improve their effectiveness.
Understanding these mechanisms is crucial for anyone involved in managing online communities, ensuring they remain safe and welcoming spaces for all users.